A new horizon in healthcare
By combining sound analysis with artificial intelligence, VoiceMed detects subtle signals in the sounds produced by the vocal apparatus. when associated with either a normal biological or pathogenic state, these signals are called “vocal biomarkers”.
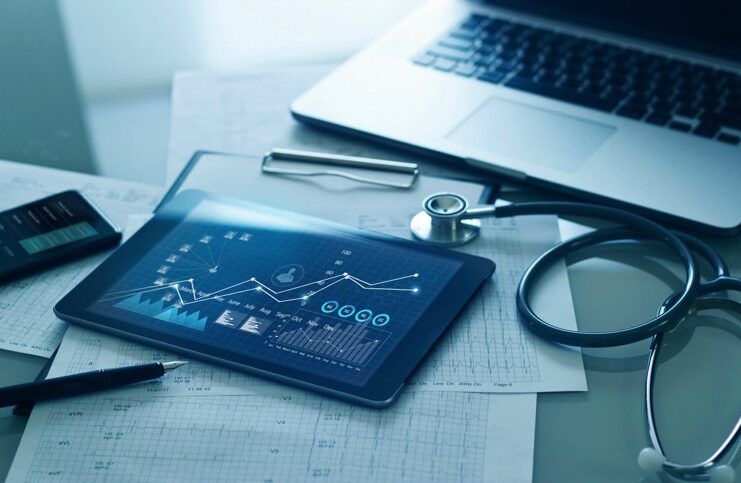
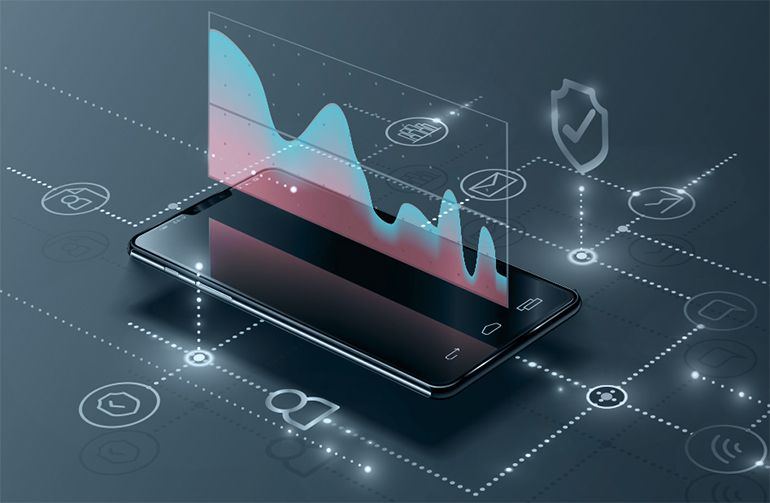
What is a vocal biomarker?
A vocal biomarker is a specific feature of any sound produced by the vocal apparatus (for example, speech, breathing sounds or cough) that indicates a specific underlying physiological and/or pathophysiological condition.
How to measure lung and fitness from vocal biomarkers?
In respiratory analysis, our lungs generate sounds that can be perceived even from forced breathing exhalation. Our body is composed of tubes, and when there is a constriction or obstruction, the air that gets in produces different volumes and sounds.
In cardiorespiratory analysis, we exploit the main and peripheral blood circulation activity around all those components involved in phonation. Indeed, lungs, diaphragm, vocal folds, tongue, and other elements perceive each heartbeat thanks to the blood flow irrigating them. this means that part of the cardiac information is transduced into the acoustic production.
Our vocal biomarkers factory
Source data
The input is composed by breathing sound recording + health label (e.g. lung function). The acoustic variations are analysed and summarized to become vocal features.
Biomarker candidates
These vocal features are interpreted by a self-learning AI system. The results of the process is a new set of parameters that summarize and generalize the biological problem: vocal biomarker candidates.
Vocal biomarkers
The outputs generated from the previous steps have a clinical interpretation and are then validated against medical-grade measurements and clinical outcomes. After this step, they can be used as vocal biomarkers.
How reliable are they?
Vocal biomarkers can be extremely reliable, but this depends on the dataset. To ensure the reliability of our vocal biomarkers, VoiceMed relies on a high-quality and extensive dataset. Unlike other technologies, such as video analysis for vital signs that depend on publicly available datasets, our vocal biomarkers dataset is exclusively created by VoiceMed. The quality and robustness of this dataset are guaranteed through a combination of rigorous clinical studies and extensive real-world campaigns, providing a solid foundation for our AI models. That is why you can rely on VoiceMed reliability products.
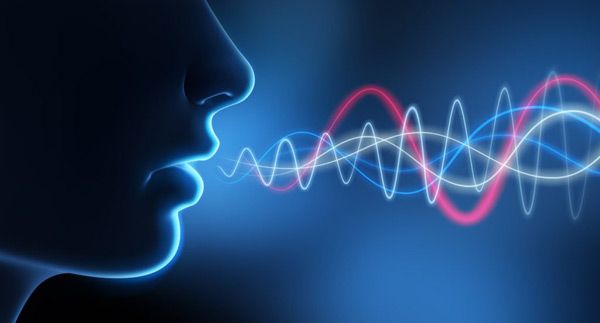
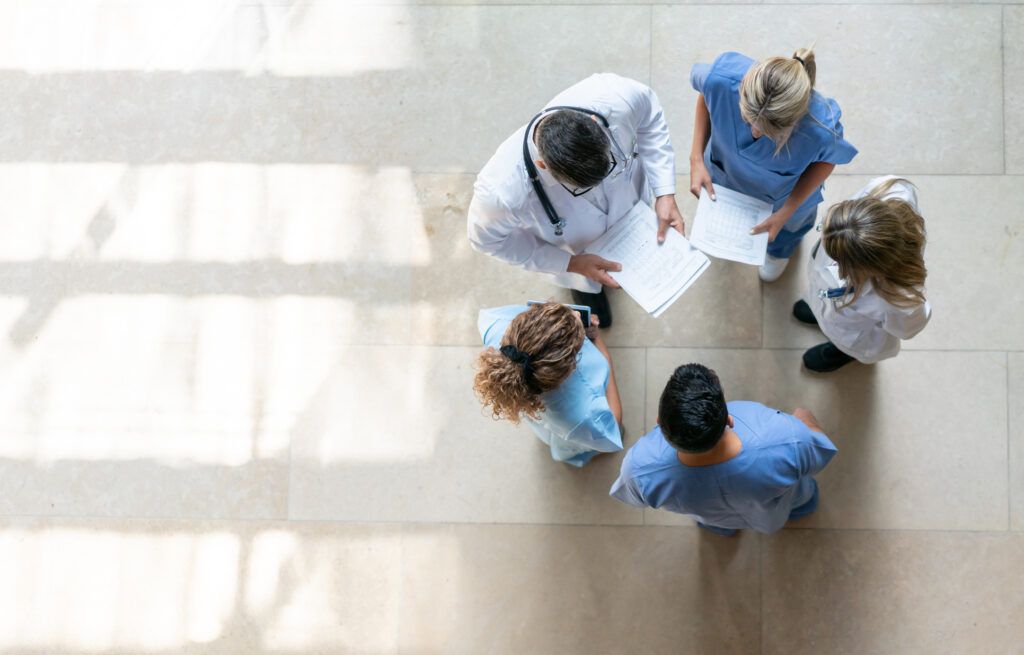
Clinical studies performed
As of 2024, we have successfully conducted three clinical studies in collaboration with prestigious European hospitals. These studies have significantly contributed to the creation of VoiceMed’s proprietary Biobank of Human Sounds, ensuring our technology is backed by robust clinical evidence. This extensive dataset enhances the accuracy and reliability of our vocal biomarkers, making our health assessments trustworthy and scientifically validated.
Microphone variability
Our technology empowers anyone with a smartphone to measure their health, which naturally presents challenges related to microphone variability across different devices. We are acutely aware of these challenges and are dedicated to minimizing their impact. By continually expanding our dataset to include data from thousands of different devices, we ensure our algorithms can adapt and maintain accuracy regardless of the hardware used. This commitment to addressing variability underscores our dedication to delivering reliable health assessments in diverse real-world conditions.

Partners & investors
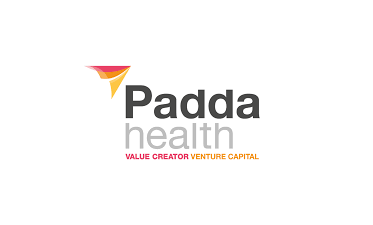
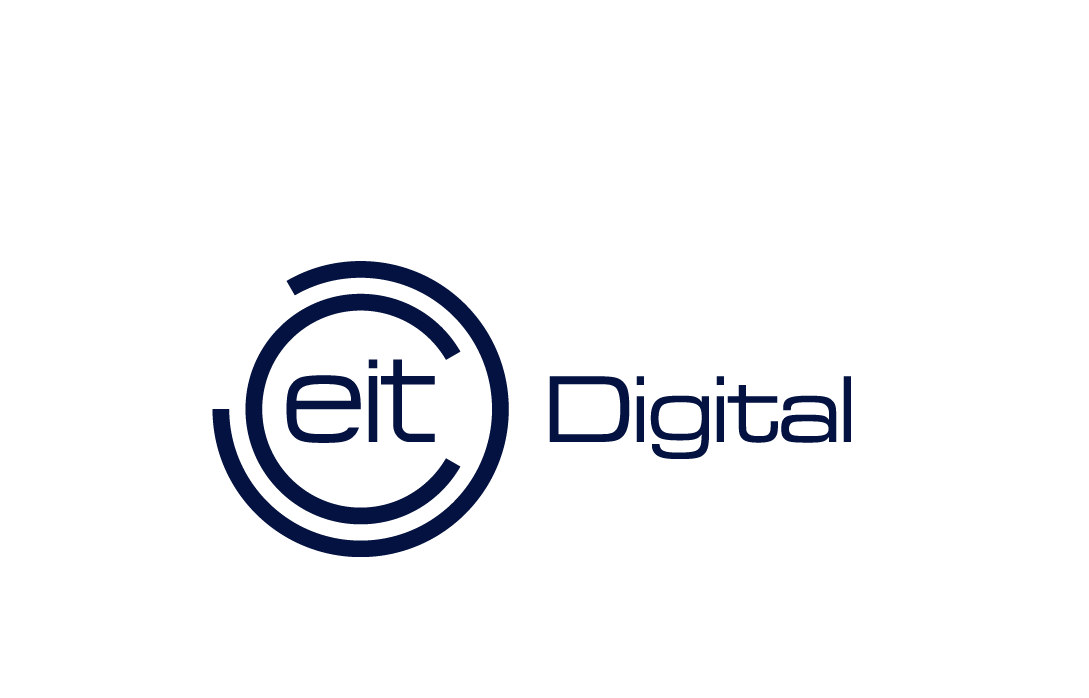
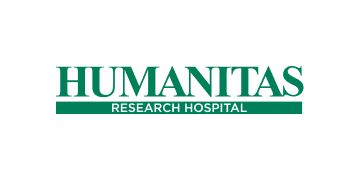
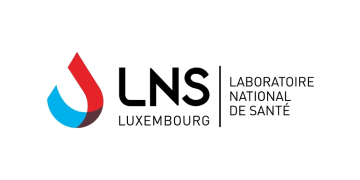
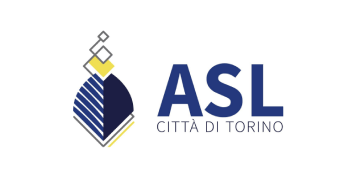
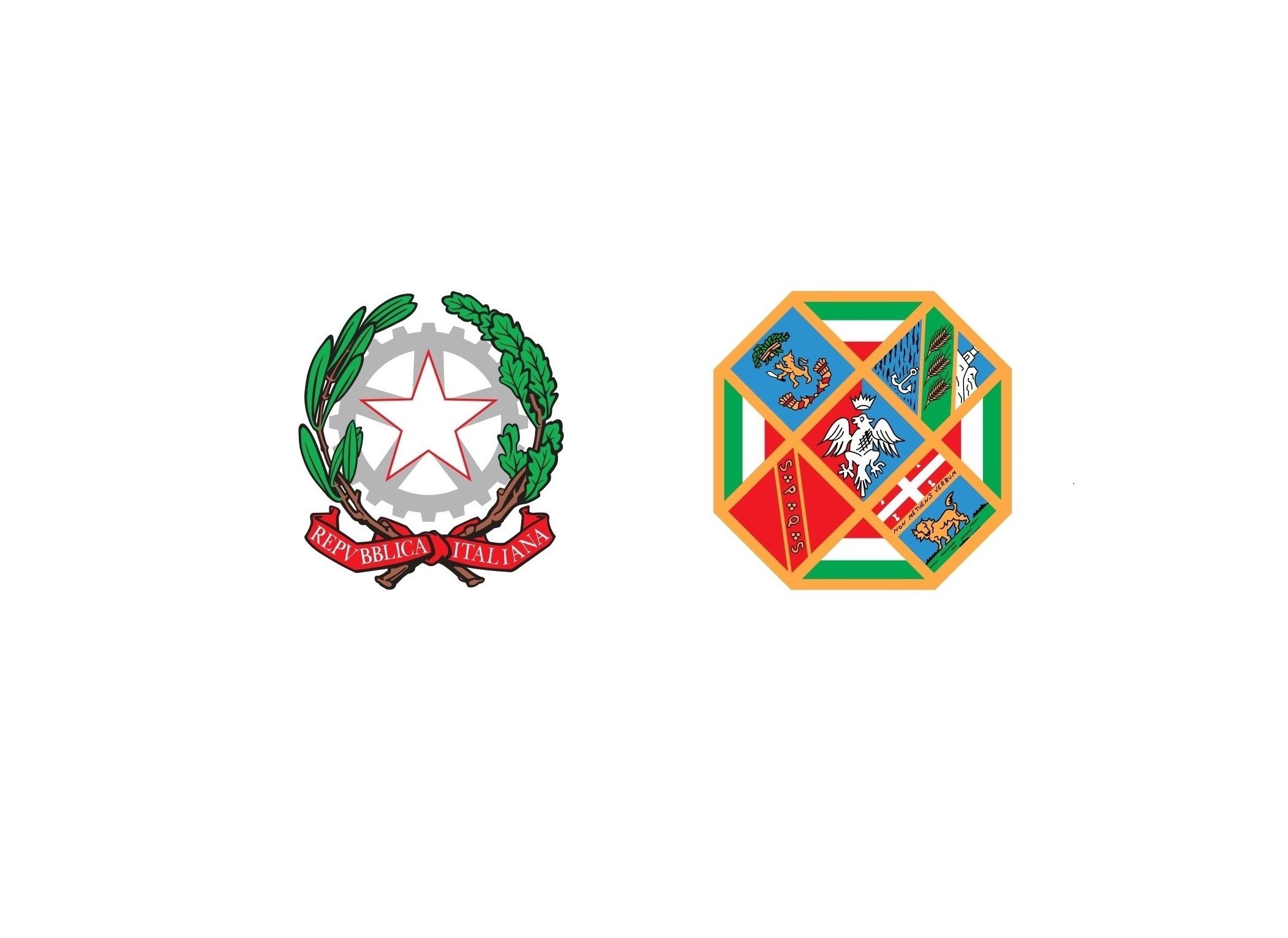
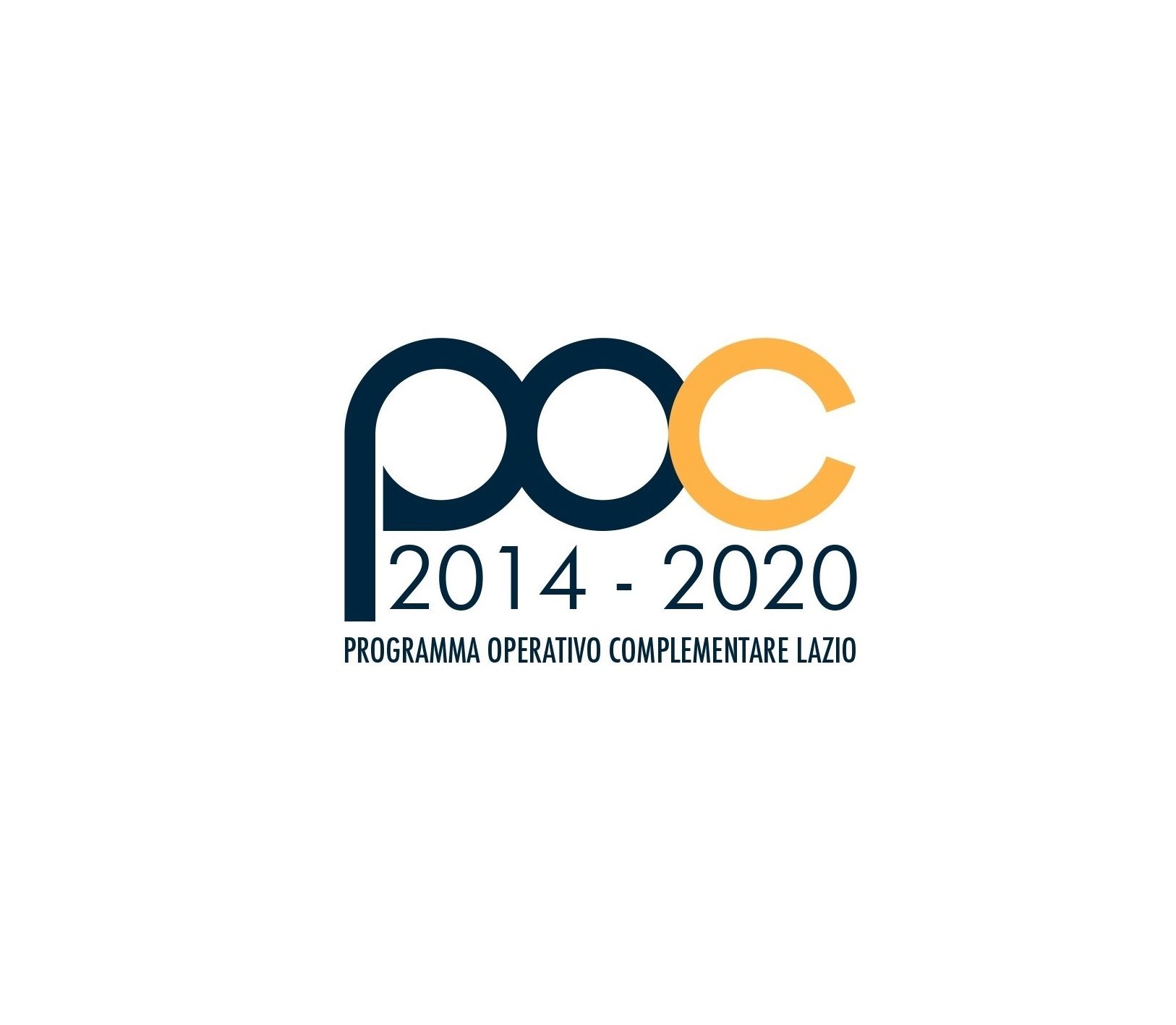
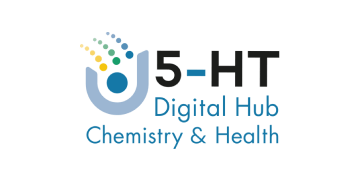
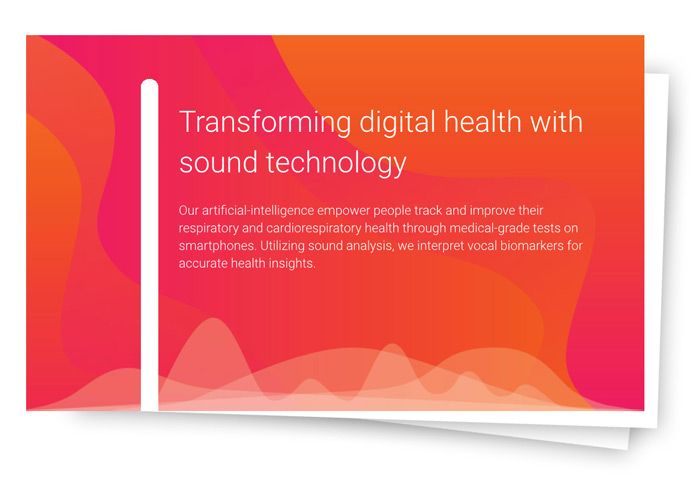
Want to know more?
Contact us to receive the white paper where you will find
more information about our technology!